While all major companies such as Google, Microsoft, and Meta have started working in the field of AI and are producing advanced AI models one after another, Apple, which is the company that remains, participated in this AI race with its multimodal large language model called MM1. This model was trained with both textual data and visual data. The MM1 multimodal large language model was trained with a mix of captioned images (45%), interleaved image-text documents (45%), and text-only (10%) data.
In this article, we will discover what Apple's MM1 multimodal large language model is.
If you're ready, let's start!
TL; DR
- MM1 is a multimodal large language model family developed and announced by Apple.
- The MM1 model was developed with a different training and building method, unlike traditional MLLMs.
- While building the MM1 model, architecture, trained data, image encoder, parameters, hyperparameters, and training procedure were re-created to be effective.
- The MM1 model has a lower number of parameters and size than its competitors, allowing it to operate smoothly on mobile devices.
- If you are looking for an AI assistant who will always be with you, such as mobile devices, TextCortex is the way to go, with its integration of 30,000+ websites and apps.
- TextCortex offers a variety of customizable and interactive AI solutions, from writing to your professional tasks.
What is Apple MM1?
Apple's MM1 is a Multimodal Large Language Model (MLLM) family that can handle and generate both text and visual processes. According to Apple's article, the working principle of the MM1 model enables AI to better understand user prompts and generate the desired output. New methods were used while training the MM1 model, and these methods claim that image resolution and the capacity of the visual encoder had the highest impact on model performance.
Is Apple falling behind the AI race?
Apple's research lab aimed to build a high performance multimodal large language model (MLLM) by carefully examining tons of AI training and building methods. According to Apple's article, using different types of data when training an AI model, using image-text documents in few-shot learning, using captioned images to increase zero-shot performance, and text-only data emphasizes that strong language understanding is important to improve performance.
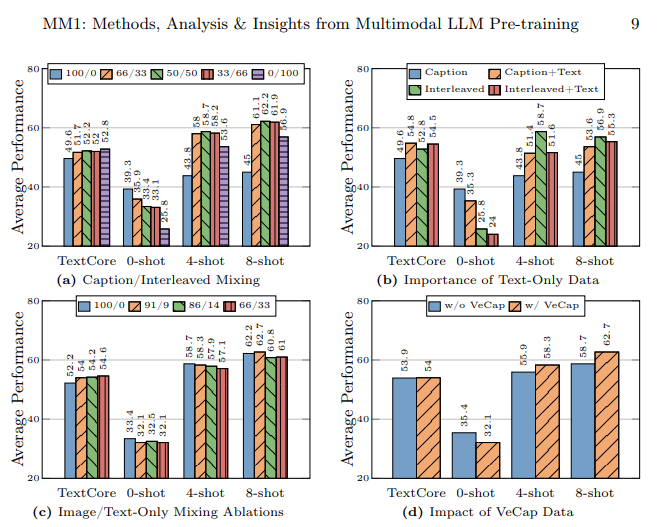
How was the MM1 Model Built?
Apple mentioned that it went beyond traditional methods to build the MM1 model and that these methods were necessary to build a high-performance Multimodal Large Language Model (MLLM). Apple considers three basic steps when building the MM1 model:
- Architecture: Apple's research lab team examined methods of connecting different pre-trained image encoders and LLMs with these encoders to build a unique architecture.
- Trained Data: The MM1 model is trained with datasets containing different types of data, such as visual and text, and combinations of these data.
- Training Procedure: Instead of being trained with a large number of parameters, the MM1 model was trained by focusing on hyperparameters and at what stage they will be used.
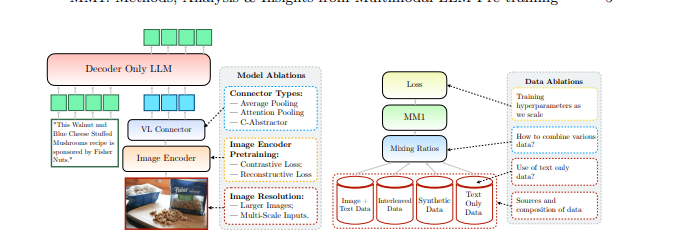
Apple’s MM1 Capabilities
Apple's MM1 Multimodal Large Language Model (MLLM) has different training methods, fewer parameters, and different approaches to the training process compared to traditional MLLMs. Apple states that the MM1 model was built by discovering the most efficient methods and trying innovations to train an MLLM. Let's take a closer look at the capabilities and training differences of the MM1 model.
Architecture
The MM1 model has a different architecture compared to other MLLMs (Multimodal Large Language Models). This architecture includes higher image resolution encoders, a different approach to the pre-training process, and mixing labelled data to boost the overall performance of a single prompt. Apple's research lab focuses on the importance of various data choices to train the MM1 model. In other words, the MM1 model has an architecture that aims to provide higher performance by using fewer resources than traditional MLLMs.
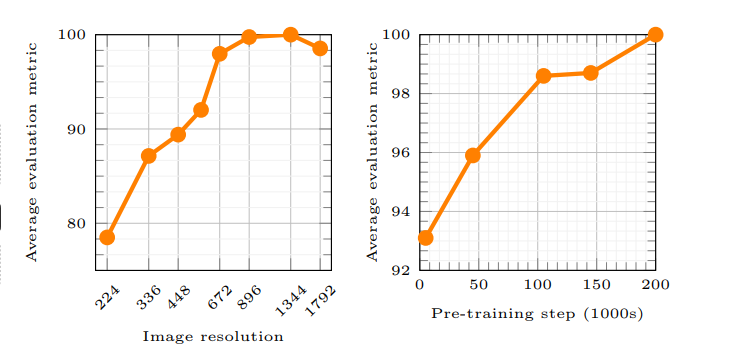
Image Encoder
Most Multimodal Large Language Models use CLIP (Contrastive Language-Image Pretraining) pre-trained image encoder to process visual data. Additionally, recent studies show that vision-only self-supervised models work more effectively. By analysing these encoders, Apple's research team discovered that the most effective method of training an MLLM with visual data is through the images selected for the encoders. According to Apple's research lab, the data used in the processing process of an image encoder will directly affect the performance of the encoder. The result of all this research is as follows: image resolution has the highest impact, followed by model size and training data composition.
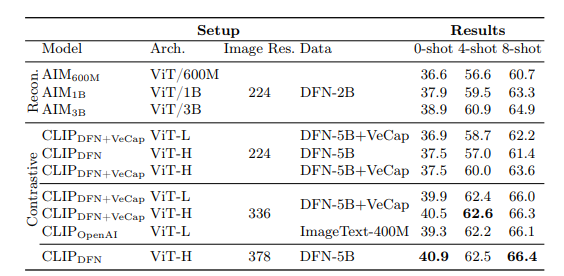
MM1 Parameters
According to Apple's research lab, what is more important than the size of parameters in a Multimodal Large Language Model (MLLM) is the fine-tuning, scaling, and hyperparameters of those parameters.
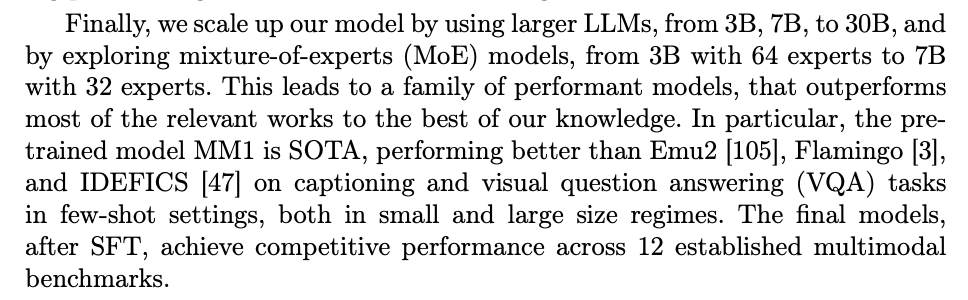
The parameters of the MM1 model were used with maximum efficiency to analyse and process various data inputs. The MM1 model is a family of AI models consisting of three different parameter sizes: 3B, 7B, and 30B. Additionally, the smaller the parameter size of an MLLM, the more efficiently it will work on mobile devices.
If you are looking for an AI assistant that will always support you on both mobile devices and desktops to help you complete your daily or professional tasks, you should keep TextCortex on your radar.